In the dynamic and ever-changing realm of data analytics, enterprises, and institutions necessitate dependable instruments for the effective processing and analysis of data. The growing significance of data-driven decision-making has prompted the emergence of many data science tools, each possessing distinct merits and drawbacks.
Read on to explore the realm of data science tools, examining the advantages and disadvantages that might significantly impact one’s analytical pursuits.
Navigating the Advantages and Disadvantages of Data Science Tools
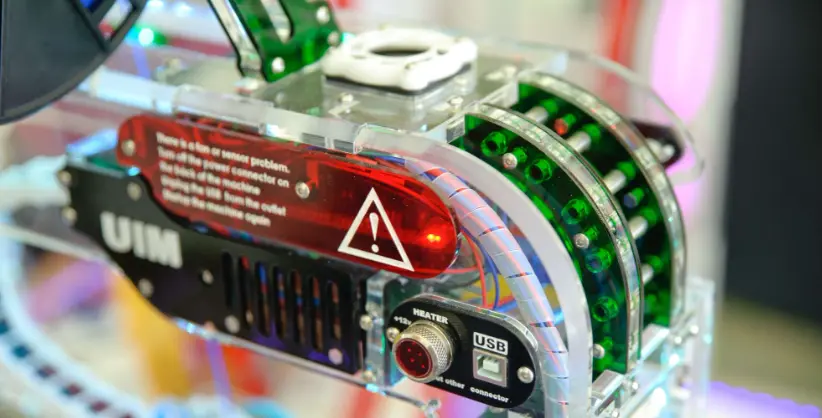
When it comes to data analytics, the right tools can make all the difference. In today’s data-driven world, businesses and organizations rely heavily on data science tools to unlock meaningful insights from their vast volumes of raw data.
These tools serve as the backbone of the analysis process, enabling professionals to process, manipulate, visualize, and interpret data, ultimately aiding in informed decision-making and strategy formulation. However, it’s essential to recognize that no tool is without its drawbacks. Let’s dive deeper into the pros and cons of data science tools.
Pros of Data Science Tools: Enhancing Analytics and Decision-Making
- Efficiency: Data science tools streamline the data analysis process, significantly reducing the time it takes to transform raw data into meaningful insights. This efficiency is especially crucial when dealing with large datasets that would otherwise require a considerable amount of manual effort.
- Insight Generation: These tools provide the means to uncover hidden patterns, correlations, and trends within the data. This insight generation allows businesses to make informed decisions based on a deeper understanding of their operations, customers, and market trends.
- Scalability: Many data science tools are designed to handle large-scale data processing. This scalability ensures that as your organization grows, the tools can accommodate increased data volumes without compromising performance.
Rather than being adversely affected by performance bottlenecks or slowdowns, these solutions are specifically intended to effectively manage the augmented data loads in a smooth and efficient manner.
- Accuracy: Advanced algorithms within these tools can enhance the accuracy of predictions and analyses, leading to more reliable results. This accuracy is particularly important when making critical business decisions.
The process reduces ambiguity, improves comprehension of intricate data patterns, and provides stakeholders with a robust basis for making strategic decisions.
The meticulous analysis and interpretation of data not only enhance the effectiveness of decision-making processes but also confer a competitive advantage by mitigating the potential hazards linked to erroneous forecasts.
- Security: Modern data science tools often come with robust security features to protect sensitive data throughout the analysis process. This ensures compliance with data privacy regulations and maintains the integrity of the data.
Access controls and authentication mechanisms further fortify the data’s confines, ensuring only authorized personnel can engage with it. Audit trails and logging functionalities offer a transparent record of interactions, promoting accountability and traceability.
Cons of Data Science Tools: Considering the Limitations
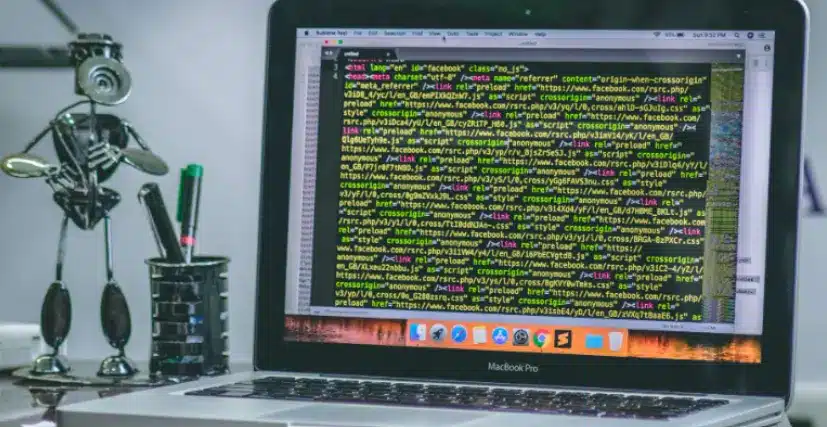
- Learning Curve: While these tools offer powerful capabilities, mastering them may require a significant investment of time and effort. This learning curve can hinder immediate adoption and implementation.
To effectively utilize these tools, individuals might need to allocate time to studying user manuals, participating in training sessions, watching tutorials, and experimenting through trial and error. Nonetheless, this initial investment of effort and time can lead to long-term proficiency, enabling users to leverage the tools’ capabilities to their fullest potential.
- Cost: Some high-quality data science tools come with a substantial price tag, making them less accessible for small businesses or individual analysts. However, there are also free or open-source options available that can still deliver valuable results.
- Dependence on Data Quality: The accuracy and reliability of the insights generated heavily depend on the quality of the input data. If the data has quality issues or inaccuracies, the tool’s output may be compromised. Investing effort in identifying and rectifying data quality problems not only enhances the reliability of the tool’s insights but also ensures that the subsequent decision-making process is well-informed and dependable.
- Resource Intensive: Analyzing data on a large scale can sometimes be resource-intensive, requiring substantial computing power and storage. This can lead to increased costs for infrastructure and maintenance.
Understanding and accounting for the resource-intensive nature of large-scale data analysis is crucial for informed decision-making, effective project planning, and successful implementation of data-driven initiatives.
Selecting the Right Tool for Your Analytical Goals
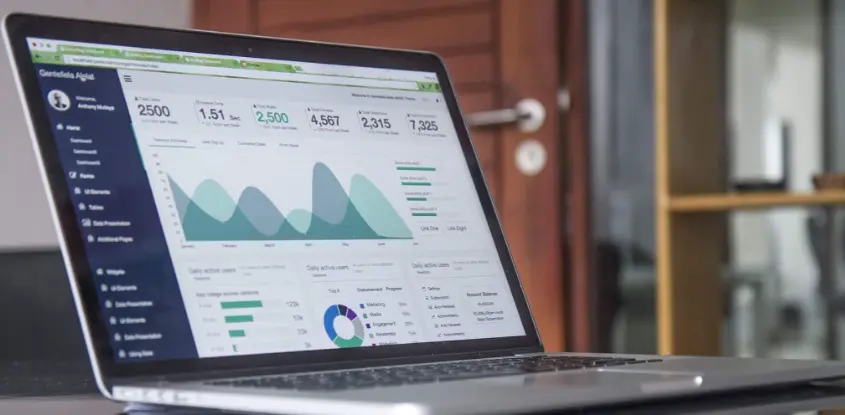
When deciding on a data science tool for your organization, it’s essential to weigh the pros and cons to make an informed choice that aligns with your business goals. Consider the following factors:
- Nature of Analysis: Different tools cater to various types of analysis, such as statistical analysis, machine learning, or visualization. Choose a tool that aligns with your specific analytical needs. It’s essential to consider factors like the complexity of the analysis, the size of the dataset, the required level of automation, and the specific goals of the project. The user’s familiarity with the tool’s interface and functionality is crucial for efficient utilization.
- Available Resources: Evaluate the resources, both in terms of budget and expertise, that you can allocate to using and maintaining the chosen tool. In terms of budget, this involves estimating the costs associated with acquiring the tool, any licensing fees, implementation expenses, ongoing maintenance, and potential training for your team to effectively use it.
Additionally, considering scalability and future upgrades is important to ensure long-term financial feasibility. Regarding expertise, assess whether your existing team possesses the necessary skills to operate and manage the tool. If the required expertise is lacking, you may need to allocate resources for training or hiring individuals with the appropriate skill set.
- Data Volume: If you’re dealing with large datasets, prioritize tools that offer scalability and efficient processing capabilities. Consider tools with optimized algorithms for tasks such as data transformation, aggregation, and analysis. Streamlining these operations can significantly reduce processing times. Compression techniques can also help mitigate the storage and processing demands of large datasets.
- Ease of Use: Consider the learning curve associated with the tool. Sometimes, the most powerful tools might be challenging to master quickly.
Allocate resources for training, onboarding, and support. This could involve tutorials, documentation, online courses, or even seeking assistance from experienced users. Prioritize tools that offer comprehensive learning resources and active user communities, as these can greatly assist in reducing the learning curve.
It’s worth noting that the learning curve isn’t necessarily a negative factor. Mastery of a sophisticated tool can lead to substantial benefits in terms of productivity, efficiency, and the ability to handle complex tasks. However, it’s important to balance the potential advantages with the time and effort required for training and skill development. - Integration: Ensure that the chosen tool can integrate seamlessly with your existing data sources, pipelines, and other software. Integration involves the smooth exchange of data and information between different tools, systems, or applications. A tool that integrates well with your existing ecosystem can prevent data silos, reduce manual data transfer efforts, and enhance overall productivity.
Making Informed Data-Driven Decisions
In the ever-expanding realm of data analytics, selecting the right data science tool is crucial for organizations aiming to leverage their data effectively. Understanding the pros and cons of these tools empowers businesses to make informed choices that align with their analytical goals. Whether it’s enhancing efficiency, uncovering hidden insights, or scaling data processing capabilities, the right tool can significantly impact the quality of analysis and decision-making.
Remember that there’s no one-size-fits-all solution. The choice of data science tool depends on factors like the nature of the analysis, available resources, and the organization’s specific needs. Don’t rush in your decision-making.
By carefully evaluating the advantages and disadvantages, you can find the perfect balance between functionality, efficiency, and cost-effectiveness, ultimately harnessing the power of data analytics to drive your business forward. Embrace the opportunity to broaden your knowledge by perusing our diverse selection of articles, and unlock a wealth of information waiting to be discovered. Visit our blog now.
Best Data Science Tools
Frequently Asked Questions
What is data science?
Data science is a multidisciplinary field that uses scientific methods, processes, algorithms, and systems to extract knowledge and insights from structured and unstructured data.
How can data analytics help in business decision-making?
Data analytics can help in business decision-making by providing valuable insights and patterns from large datasets. It enables businesses to identify trends, understand customer behavior, optimize operations, and make data-driven strategic decisions.
What are the pros of using data science tools?
Using data science tools has numerous advantages, including:
– Ability to analyze large volumes of data quickly and efficiently.
– Identification of patterns and trends that may not be obvious to humans.
– Enhancement of decision-making processes through data-driven insights.
– Automation of repetitive tasks, saving time and resources.
– Creation of predictive models that help in forecasting future outcomes.
What are the cons of using data science tools?
While data science tools offer many benefits, there are also some drawbacks to consider:
– Complexity: Data science tools often require specialized knowledge and expertise to operate effectively.
– Time-consuming: Performing data analysis can be a time-consuming process, especially when dealing with large datasets.
– Potential bias: Data science tools can be influenced by biased data, leading to inaccurate results and decisions.
– Costly: Some data science tools require a significant investment in terms of licensing, infrastructure, and training.
– Limited interpretability: The output of data science models can sometimes be difficult to interpret and explain.
What is a data analytics platform?
A data analytics platform is a software solution that provides tools and capabilities for collecting, analyzing, and visualizing data. It typically offers functionalities such as data integration, data exploration, data modeling, and reporting.
How can data analytics platforms help in identifying data patterns?
Data analytics platforms use advanced algorithms and techniques to identify patterns and relationships within large datasets. These platforms can analyze data from various sources, apply statistical methods, and generate visualizations that highlight data patterns.
Can data science tools be used in enterprise management?
Yes, data science tools can be used in enterprise management to support decision-making processes, optimize operations, and improve overall business performance. They can help in areas such as risk management, supply chain optimization, customer segmentation, and fraud detection.
Are there any use cases where data science tools have been particularly beneficial?
Yes, there are many use cases where data science tools have shown great potential. Some examples include:
Healthcare: Data science tools have been used to improve patient outcomes, predict disease outbreaks, and optimize resource allocation.
Retail: These tools have been utilized to analyze customer behavior, optimize pricing strategies, and personalize marketing campaigns.
Finance: Data science tools help in detecting fraudulent transactions, predicting market trends, and optimizing investment portfolios.
Manufacturing: These tools enable predictive maintenance, quality control, and supply chain optimization.
Social media: Data science tools analyze user behavior, sentiment analysis, and personalized recommendations.
How can data science tools assist in monitoring and improving performance?
Data science tools can monitor and analyze key performance indicators (KPIs) to assess the current state of operations. They can identify areas of improvement, provide insights into bottlenecks, and enable data-driven decision-making to drive performance enhancements.
Is it necessary to have prior data science knowledge to use these tools?
While having prior data science knowledge can be beneficial, many data science tools are designed with a user-friendly interface that allows users with limited technical knowledge to leverage their functionalities. However, a basic understanding of data science concepts and principles can still be advantageous.